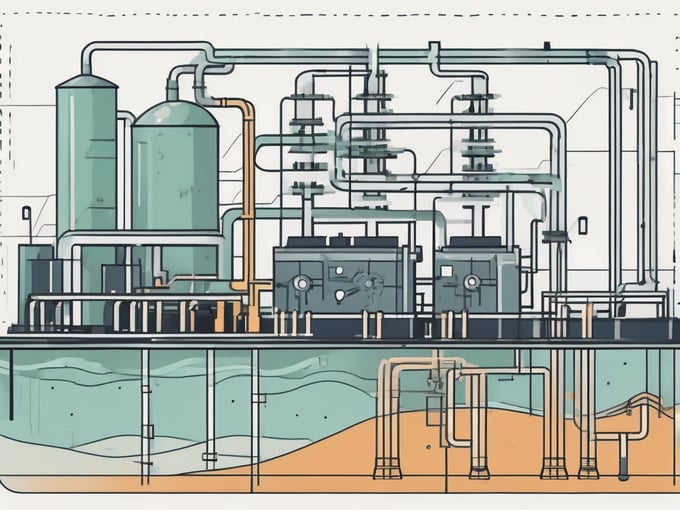
Important Trends in Data Analytics for Plant Operators in the Sludge Management Industry
In today's rapidly evolving sludge management industry, the role of data analytics has become increasingly important. Plant operators are now realizing the potential of harnessing data to optimize their operations, improve efficiency, and make informed decisions. This ultimate guide will delve into the world of data analytics, exploring its significance in sludge management and providing key insights on its implementation.
Understanding the Importance of Data Analytics in Sludge Management
In order to comprehend the true value of data analytics in sludge management, it is crucial to understand its role in plant operations. Data analytics plays a pivotal role in enabling operators to gain deeper insights into their processes, identify trends, and identify areas for improvement. By harnessing the power of data, operators can enhance their decision-making and strategically plan for the future.
The Role of Data Analytics in Plant Operations
Data analytics empowers plant operators to optimize their operations by providing real-time visibility into the sludge management processes. From monitoring key parameters to detecting anomalies, data analytics enables operators to stay informed and promptly address any issues that may arise. By leveraging data analytics, operators can streamline their operations and ensure efficient sludge management.
Furthermore, data analytics allows operators to analyze historical data to identify patterns and trends. This information can be used to make informed decisions about process adjustments, resource allocation, and risk management. By understanding the historical performance of the sludge management system, operators can proactively address potential issues and optimize their processes for maximum efficiency.
In addition, data analytics can also be used to monitor the performance of individual equipment within the sludge management system. By collecting and analyzing data from sensors and monitoring devices, operators can identify equipment that may be underperforming or in need of maintenance. This proactive approach to equipment management can help prevent costly breakdowns and ensure that the sludge management system operates at its full potential.
Benefits of Implementing Data Analytics in Sludge Management
The benefits of implementing data analytics in sludge management are manifold. Firstly, operators can improve operational efficiency by identifying areas that require optimization. By analyzing data on sludge production, treatment efficiency, and energy consumption, operators can identify bottlenecks and inefficiencies in the system. This knowledge allows them to make targeted improvements, such as adjusting process parameters or implementing new technologies, to increase overall efficiency.
Secondly, data analytics aids in predicting future trends, enabling proactive decision-making and reducing downtime. By analyzing historical data and using predictive modeling techniques, operators can anticipate changes in sludge production rates, treatment requirements, and equipment performance. This foresight allows them to plan ahead, allocate resources effectively, and minimize disruptions to the sludge management process.
Thirdly, by leveraging data analytics, operators can enhance asset management, ensuring that equipment is optimized and maintenance is carried out effectively. By monitoring equipment performance and analyzing data on maintenance activities, operators can identify opportunities for preventive maintenance and optimize the scheduling of maintenance tasks. This proactive approach can extend the lifespan of equipment, reduce the risk of unexpected failures, and ultimately save costs associated with equipment replacement.
In conclusion, data analytics plays a crucial role in sludge management by providing operators with valuable insights, optimizing plant operations, and improving overall efficiency. By harnessing the power of data, operators can make informed decisions, predict future trends, and enhance asset management. Implementing data analytics in sludge management is not only beneficial for the operators but also for the environment, as it allows for more sustainable and effective sludge treatment processes.
Key Concepts in Data Analytics for Plant Operators
For plant operators new to data analytics, it is essential to grasp some fundamental concepts. Data mining and analysis form the backbone of data analytics, enabling operators to extract valuable information and uncover patterns. Predictive analytics, on the other hand, utilizes historical data to predict future outcomes, allowing operators to anticipate potential issues and plan accordingly.
Introduction to Data Mining and Analysis
Data mining involves using algorithms to analyze vast amounts of data and extract meaningful patterns or relationships. By identifying trends and correlations, operators can gain valuable insights into their sludge management processes, enabling them to make data-driven decisions and improve overall efficiency.
When it comes to data mining, operators must first understand the different techniques available. These techniques include classification, clustering, association rule mining, and anomaly detection. Classification involves categorizing data into predefined classes or groups based on specific attributes. Clustering, on the other hand, involves grouping similar data points together based on their similarities and differences. Association rule mining focuses on discovering relationships between different variables in the dataset, while anomaly detection identifies unusual or abnormal data points that deviate from the norm.
Once operators have a good understanding of the data mining techniques, they can start applying them to their plant operations. For example, in sludge management, operators can use classification algorithms to categorize different types of sludge based on their characteristics. This can help them determine the most appropriate treatment methods for each type, leading to improved efficiency and cost savings.
Predictive Analytics and Its Application in Plant Operations
Predictive analytics utilizes historical data and statistical techniques to forecast future outcomes. For plant operators, this means being able to anticipate potential issues, such as equipment failures or sludge quality fluctuations. By leveraging predictive analytics, operators can plan preventive maintenance and make informed decisions to mitigate risks.
There are several predictive modeling techniques that plant operators can use to analyze their data. These techniques include regression analysis, time series analysis, and machine learning algorithms. Regression analysis helps identify relationships between variables and predict future values based on historical data. Time series analysis, on the other hand, focuses on analyzing data points collected over time to identify patterns and trends. Machine learning algorithms, such as decision trees or neural networks, can be used to build predictive models that can make accurate predictions based on historical data.
Once operators have built their predictive models, they can use them to forecast various aspects of their plant operations. For example, they can predict the future sludge production based on historical data, allowing them to plan their storage and disposal strategies accordingly. They can also predict the likelihood of equipment failures based on maintenance records and sensor data, enabling them to schedule preventive maintenance activities and minimize downtime.
In conclusion, data analytics plays a crucial role in plant operations, enabling operators to extract valuable insights from their data and make informed decisions. By understanding the key concepts of data mining and analysis, as well as predictive analytics, operators can harness the power of data to optimize their processes, improve efficiency, and reduce risks.
Tools and Techniques for Effective Data Analytics
To effectively harness the power of data analytics, plant operators must familiarize themselves with the tools and techniques available. Data analytics tools such as data visualization software and data management systems can facilitate the analysis and interpretation of data. Additionally, techniques for data visualization and interpretation provide operators with a clear understanding of the insights gained from the data.
When it comes to data analytics tools, the options are vast and varied. One popular tool is statistical software, which allows operators to perform complex calculations and statistical analyses on their data. These software packages often come equipped with a wide range of functions and algorithms, making it easier for operators to uncover patterns, trends, and correlations within their datasets.
Another valuable tool in the data analytics arsenal is data visualization software. This type of software allows operators to transform raw data into visually appealing and interactive graphs, charts, and infographics. By presenting data in a visual format, operators can easily identify patterns and outliers, making it easier to communicate their findings to stakeholders and decision-makers.
Data management systems are also essential for effective data analytics. These systems provide operators with a centralized platform to store, organize, and manage their data. With features like data cleansing, data integration, and data security, operators can ensure that their data is accurate, up-to-date, and easily accessible for analysis.
Overview of Data Analytics Tools
Data analytics tools encompass a wide range of software and platforms designed to facilitate data analysis. From robust statistical software to user-friendly data visualization tools, operators can choose the tools that best suit their needs. These tools enable operators to process large datasets, generate meaningful visualizations, and extract actionable insights.
In addition to statistical software and data visualization tools, there are other specialized tools available for specific data analytics tasks. For example, machine learning algorithms and artificial intelligence (AI) tools can be used to uncover hidden patterns and make predictions based on historical data. These advanced tools are particularly useful in industries where predictive analytics plays a crucial role, such as energy management and predictive maintenance.
Cloud-based data analytics platforms are also gaining popularity among plant operators. These platforms offer the advantage of scalability and flexibility, allowing operators to analyze large volumes of data without the need for expensive hardware or infrastructure. With cloud-based analytics, operators can access their data from anywhere, collaborate with team members in real-time, and take advantage of advanced analytics capabilities.
Techniques for Data Visualization and Interpretation
Data visualization is a powerful technique that allows operators to present complex data in a visually appealing and easy-to-understand format. Through graphs, charts, and infographics, operators can communicate insights derived from data effectively. By improving data interpretation, operators can make more informed decisions and drive positive changes in their sludge management processes.
When it comes to data visualization techniques, there are several approaches that operators can employ. One common technique is the use of line charts, which are ideal for showing trends over time. By plotting data points on a line chart, operators can easily identify patterns, fluctuations, and seasonality in their data.
Bar charts are another popular visualization technique, particularly useful for comparing different categories or groups. By representing data as bars of varying lengths, operators can quickly compare values and identify outliers or anomalies. Bar charts are especially effective when dealing with discrete or categorical data.
Pie charts, on the other hand, are ideal for illustrating proportions and percentages. By dividing a circle into slices, operators can visually represent the distribution of a variable across different categories. Pie charts are particularly useful when operators want to highlight the contribution of each category to the whole.
Infographics are a more creative and visually engaging way to present data. By combining text, images, and graphics, operators can tell a compelling story and convey complex information in a concise and memorable manner. Infographics are especially useful when operators need to communicate their findings to a non-technical audience or stakeholders who may not be familiar with data analytics.
In conclusion, the tools and techniques for effective data analytics are diverse and constantly evolving. By leveraging the right tools and employing effective data visualization techniques, plant operators can unlock the full potential of their data, gain valuable insights, and make informed decisions to improve their operations.
Implementing Data Analytics in Your Plant Operations
Now that the importance of data analytics and the key concepts have been covered, it is essential to understand how to integrate data analytics into plant operations effectively.
Data analytics is revolutionizing the way plant operations are managed, providing valuable insights and driving informed decision-making. By harnessing the power of data, plant operators can optimize processes, improve efficiency, and reduce costs.
Integrating data analytics into plant operations requires a systematic approach. First and foremost, operators should identify the specific areas where data analytics can be most beneficial. This could include optimizing energy consumption, predicting equipment failures, or improving product quality. By pinpointing these areas, operators can focus their efforts and resources effectively.
Once the areas of focus have been identified, operators should ensure that the necessary data is collected, organized, and stored securely. This involves setting up data collection systems, such as sensors or monitoring devices, to gather relevant information. The data should then be organized in a structured manner, making it easily accessible for analysis.
The next step involves selecting suitable data analytics tools and techniques. There are various tools available in the market, ranging from simple data visualization software to advanced machine learning algorithms. Operators should carefully evaluate their needs and choose tools that align with their objectives and capabilities.
Furthermore, operators should establish a robust data analytics process. This includes defining clear objectives, developing analytical models, and implementing data-driven decision-making frameworks. Regular monitoring and evaluation of the process are crucial to ensure its effectiveness and make necessary adjustments.
Overcoming Challenges in Data Analytics Implementation
Implementing data analytics in plant operations is not without its challenges. However, by proactively addressing these challenges, plant operators can unlock the full potential of data analytics.
One common challenge is data integration complexities. Plant operations generate vast amounts of data from various sources, such as sensors, equipment, and production systems. Integrating this data and ensuring its compatibility can be a complex task. However, with the right data integration strategies and technologies, operators can overcome this challenge and create a unified data environment.
Another challenge is the need for skilled personnel. Data analytics requires expertise in data science, statistics, and programming. Plant operators may need to invest in training their existing workforce or hiring specialized professionals to effectively implement data analytics. Collaborating with experienced data analytics providers can also be a viable solution to bridge the skill gap.
Ensuring data quality and consistency is another critical challenge. Inaccurate or incomplete data can lead to faulty analysis and unreliable insights. Plant operators should establish data governance practices, including data validation and cleansing processes, to maintain data integrity. Implementing data quality assurance measures and regularly auditing data sources can help ensure the accuracy and consistency of the data used for analytics.
By proactively addressing these challenges and partnering with experienced data analytics providers, plant operators can overcome hurdles and unlock the full potential of data analytics. The insights gained from data analytics can drive continuous improvement, optimize operations, and ultimately contribute to the overall success of the plant.
Optimizing Sludge Management through Data Analytics
Data analytics presents plant operators with opportunities to optimize their sludge management processes and achieve superior operational outcomes.
Improving Efficiency and Productivity
By utilizing data analytics, plant operators can uncover inefficiencies and bottlenecks within their sludge management processes. Armed with this knowledge, operators can implement targeted improvements, streamline operations, and enhance productivity. From optimizing sludge treatment to reducing energy consumption, data analytics enables operators to make data-driven decisions for continuous improvement.
Enhancing Decision-Making and Strategic Planning
Data analytics empowers plant operators to make informed decisions based on accurate and timely insights. Whether it is choosing the optimal sludge disposal method or planning for future capacity expansions, data analytics provides operators with the necessary information to make strategic choices. By leveraging data analytics, operators can align their sludge management practices with long-term business goals.
Future Trends in Data Analytics for Sludge Management
As technology continues to advance, data analytics in the sludge management industry is poised for even greater advancements.
The Role of Artificial Intelligence and Machine Learning
Artificial intelligence (AI) and machine learning (ML) are revolutionizing data analytics in sludge management. By leveraging AI and ML algorithms, plant operators can automate data analysis processes, detect anomalies in real-time, and predict sludge properties with greater accuracy. This enables operators to optimize their operations and make data-driven decisions, leading to improved efficiency and sustainability.
The Impact of Big Data and IoT in Sludge Management
The emergence of big data and the Internet of Things (IoT) is transforming the sludge management landscape. With an increasing amount of sensor data collected from IoT devices, operators now have access to a wealth of real-time information. By integrating this data with advanced analytics, operators can gain deeper insights into their processes and further enhance their sludge management practices.
In conclusion, data analytics is revolutionizing the sludge management industry by enabling plant operators to optimize their operations, improve efficiency, and make informed decisions. By understanding the importance of data analytics, grasping key concepts, and utilizing effective tools and techniques, operators can unlock the full potential of their sludge management processes. With the future trends of artificial intelligence, machine learning, big data, and IoT, data analytics will continue to play a pivotal role in driving innovation and sustainability in the sludge management industry.